Unlocking AI's Full Potential in Enterprise Transformation
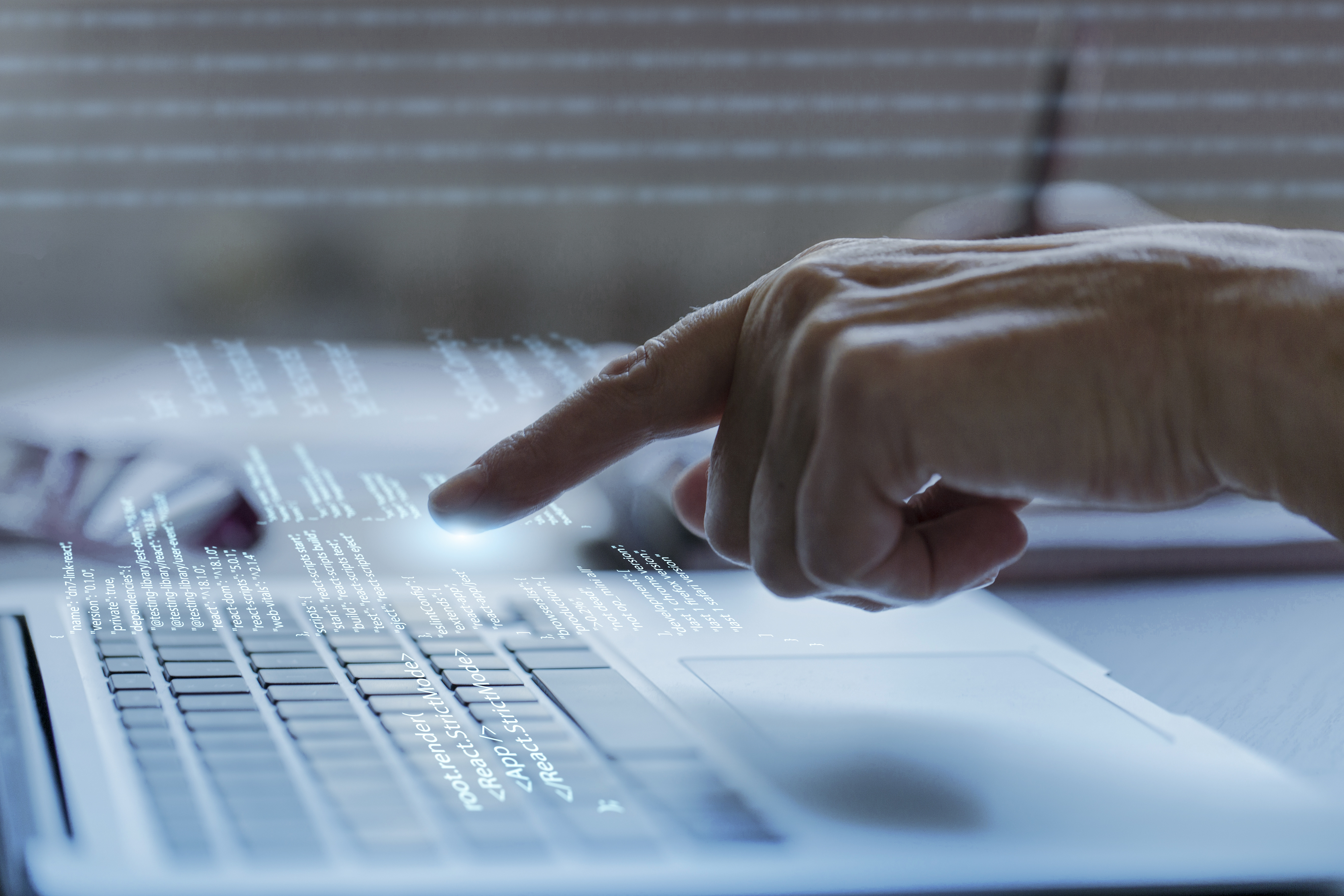
Discussion with Soumya Ganguly
With over two decades of experience in the tech services sector, Soumya Ganguly has led enterprise data initiatives across global banks and financial institutions, helping organizations modernize legacy systems and build data-driven cultures. Drawing from his cross-continental experience in India, Canada, and the United States, Ganguly now spearheads advanced analytics for a data-centric organization at the forefront of transformation. In this article, he explores how enterprises can align data and AI strategies with business goals, the operational and cultural shifts required, and real-world use cases that demonstrate the power of AI when it's paired with the right infrastructure and mindset.
Why Data and AI Are Now Business Imperatives
Today’s complex economic climate—with volatile interest rates, trade restrictions, and intensifying competition—demands that organizations operate with both speed and precision. Data and AI have emerged as not just tools, but as fundamental enablers of this shift. "Data is the digital representation of the business", Ganguly explains. "When you digitize a business process, the data captured becomes a mirror of the operation itself. If you can read that data, you can understand and improve your business".
AI amplifies this potential, enabling organizations to scale personalized training, automate processes, optimize workflows, and even drive creativity. For example, generative AI can be leveraged to develop new products or enhance services through automated experimentation and ideation—functions that were once deeply manual. But perhaps more importantly, AI delivers impact on both the top and bottom lines: increased efficiency, faster decision-making, and higher customer engagement. "Organizations that operate based on insights from data will outperform those that rely on instinct or tradition", Ganguly notes. The shift isn’t optional—it’s existential.
Key Challenges in Enterprise AI and Data Adoption
Despite growing interest and investment, many organizations struggle to adopt AI and data strategies effectively. Ganguly points to four critical obstacles:
The number one challenge he mentions, that is a critical first step for the others to follow and work is the trust in your data. "If your organization doesn’t believe in the dashboard or insights you present, they won't act on it".
The second issue is a lack of AI-ready data. While most companies collect information suitable for reporting, very few maintain the quality, structure, or consistency required for AI model training or real-time analytics. The third hurdle is limited enterprise-wide adoption—data initiatives are often confined to IT or analytics departments, rather than embedded across functions. And fourth, manual processes still dominate in many firms. "Too many organizations still run on Excel, we need to move from manual data handling to automated pipelines that are accurate, real-time, and scalable", Ganguly says.
These challenges are compounded by cultural resistance. Without change management and leadership buy-in, even the most sophisticated tools can fall flat. Building trust, standardizing processes, and offering consistent data access are all fundamental first steps.
Aligning Data & AI Strategies to Business Goals
To truly unlock the value of AI, organizations must tightly align data strategies to overarching business outcomes. According to Ganguly, this journey involves three core phases: automation, migration, and innovation.
"Start with automating your data flow from a single source of truth", he advises. This means eliminating manual reporting, consolidating data sources, and implementing standard governance frameworks. Doing so not only increases trust in the data but also paves the way for scale.
The second phase—migration—requires transitioning from on-premise systems to hyperscalers and cloud-native environments. This ensures that as the volume, velocity, and variety of data grow, performance doesn’t degrade. Key to this stage is embedding data quality and governance as foundational features.
"Finally comes the paradigm shift", Ganguly emphasizes. "You’re no longer just running your existing business better—you’re reimagining how business is done. That’s where generative AI comes in." By layering internal data with external, industry-specific insights, companies can identify new market opportunities, innovate on products, and fundamentally transform their operating model.
Real-World Use Cases: From Strategy to Impact
Ganguly shared three compelling case studies from his tenure as Head of Data at a major financial institution, where data and AI created tangible business value.
In one project, his team partnered with the COO to investigate underperforming operational metrics. "We found huge inefficiencies—manual processes, outdated data, and slow decision cycles", he recalls. After implementing automation and a centralized data platform, the customer satisfaction rating jumped from 61% to 87%, and the cross-sell rate more than doubled to 35%. "We also improved the operational staff-to-customer ratio from 1:10 to 1:22", Ganguly adds, highlighting the scale of impact.
In another initiative, his team supported the M&A division by analyzing the profitability and market footprint of various business lines. Their insights led to the strategic divestiture of a profitable but stagnant health insurance arm, which was successfully sold to a better-positioned competitor. "It was a win-win. We monetized the asset and the acquiring firm gained market share".
The third case involved building a treasury services pricing model. Using AI, the team forecasted client volumes based on market comparables and optimized pricing accordingly. "This allowed our treasury sales team to offer more aggressive, tailored pricing strategies—leading to better retention and new sales".
Fostering a Data-Driven Culture
Beyond tools and infrastructure, cultural transformation is essential. "The Chief Data Officer is now on par with CIOs and CFOs in many firms", Ganguly notes, citing Procter & Gamble as a prime example, where the CDO reports directly to the CEO.
Ganguly recommends three steps to enable a data-first culture:
- Enforce usage of centrally governed, high-quality data.
- Implement robust technical and business data quality processes.
- Build self-service environments where business users can directly access and analyze data.
When everyone in the organization is aligned with this mindset, data becomes an asset—not a challenge. "This removes bottlenecks and empowers teams, no more waiting weeks for IT to deliver a dataset. Everyone should be able to explore insights independently".
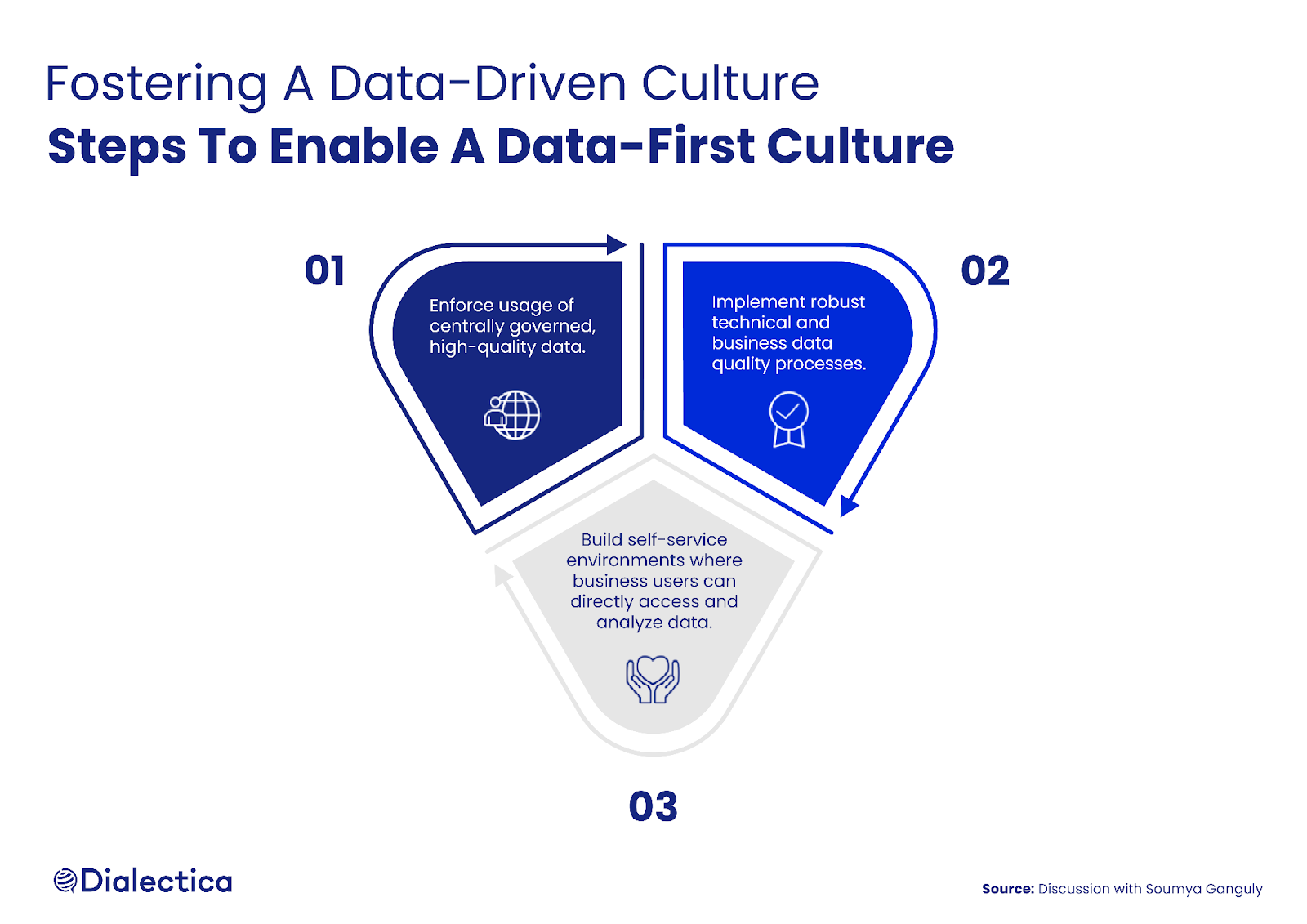
Measuring ROI in a Long-Term Transformation
One of the most visible ways to measure return on investment from data and AI initiatives is by looking at productivity gains. Ganguly explains that when companies automate manual processes, they can redeploy human capital to higher-value activities. For instance, if a task previously required 50 people but automation enables the same task to be handled by 30, the remaining 20 can be redirected toward innovation, customer engagement, or strategic planning—thereby increasing the organization’s overall value output.
Another method Ganguly highlights is through the monetization of data assets. This involves actively tracking who uses the data, how often, and for how long. By attaching metrics to these behaviors, organizations can gain visibility into how essential their data platforms have become. If usage grows from 100 to 500 users over a period of time, it’s a clear indication that the platform is generating value and contributing to operational efficiency.
Finally, API-ification—or the practice of making data accessible via APIs—plays a crucial role. When data is made available internally and externally through APIs, it becomes far more dynamic and scalable. Moreover, because API usage can be measured, organizations can monitor demand in real time. This ability to track and quantify usage allows companies to correlate data accessibility with improved outcomes, be it in the form of sales, engagement, or time saved. "When your data usage goes from 100 to 500 people—and they’re using it daily—you know you’ve created a valuable asset", Ganguly says.
Looking Ahead: Generative AI’s Expanding Role
Ganguly sees generative AI transforming enterprise functions in multiple significant ways. One of the most immediate areas of impact is training. By enabling personalized learning experiences and tailored feedback mechanisms, generative AI supports workforce development at scale. This means companies can upskill employees faster and more effectively, ultimately driving better performance across departments.
Another major impact is on operational efficiency. With generative AI, repetitive tasks and routine processes can be automated, freeing up human capital for more strategic activities. Ganguly points out that this leads to optimized workflows and faster execution, which are critical advantages in today’s fast-moving markets.
Creativity is also getting a boost. Generative AI enables idea generation and creative experimentation that would otherwise be limited by human bandwidth or imagination. This capability empowers companies to explore new product and service designs that differentiate them in the marketplace.
Finally, productivity sees an uplift through automated data analysis and content generation. Whether it’s generating customer reports or insights dashboards, AI tools can streamline output without compromising quality. According to Ganguly, these developments are already making an impact—but adoption must follow a structured timeline to ensure readiness, especially as many organizations are still trying to grasp the full potential of these technologies.
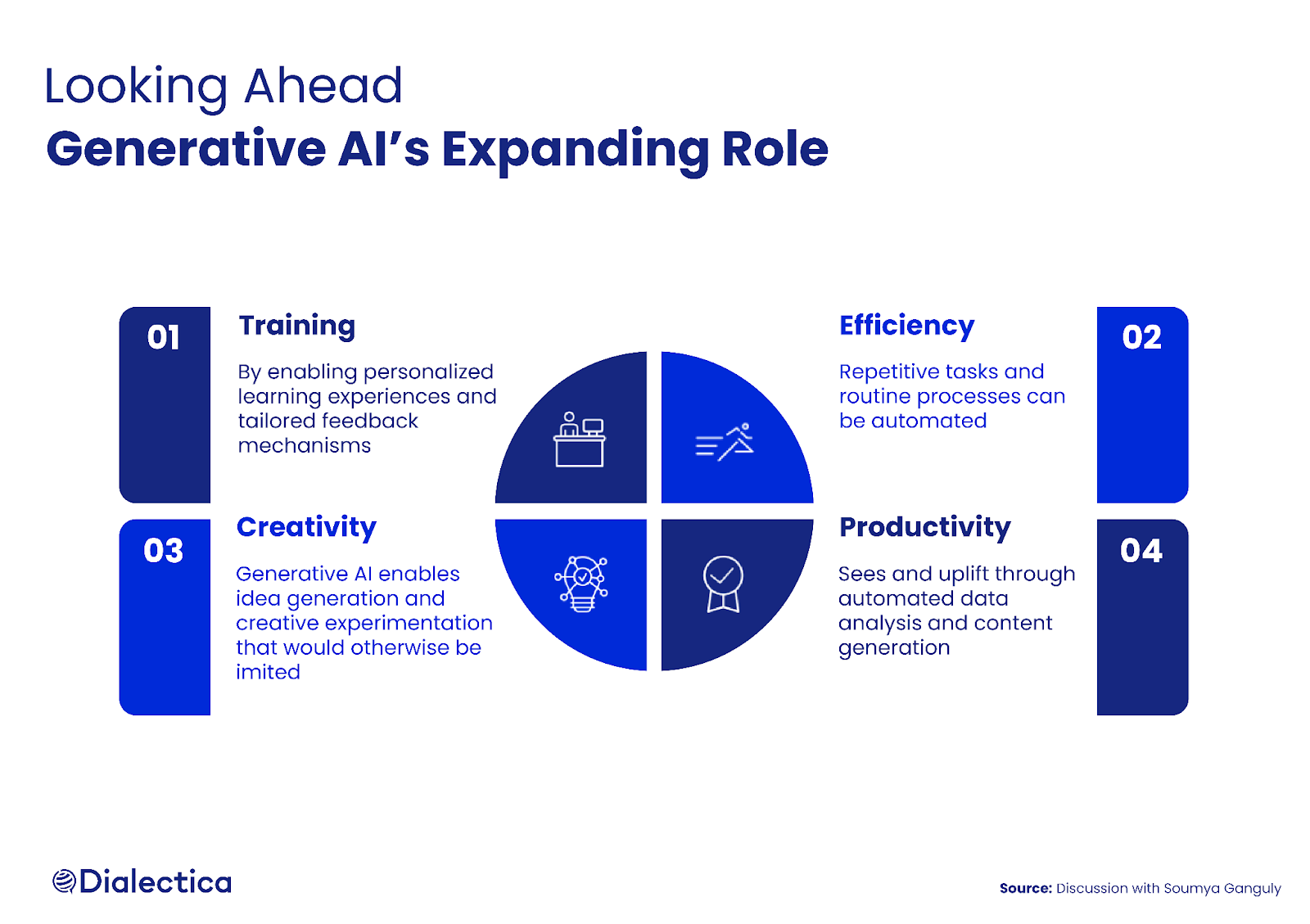
Balancing Ethics and Innovation
Ethical considerations in AI—fairness, transparency, and accountability—cannot be quantified, but they must be embedded into development. "It’s a soft skill, but a critical one," Ganguly explains. "Collaboration between developers, ethicists, and policymakers is the way forward".
He recommends integrating ethical discussions into agile ceremonies and development planning to ensure that ethics aren’t an afterthought, but a foundational pillar.
Final Takeaways for C-Level Leaders
1. AI adoption is no longer optional. Ganguly explains that many organizations, including Shopify, are beginning to require proof that AI cannot fulfill a role before allowing new hires. This signals a wider shift where companies must demonstrate the ROI of their AI capabilities before receiving further investment. Leaders must act proactively to incorporate AI into their operations or risk falling behind.
2. Sales and marketing must evolve. With high interest rates and new trading restrictions, organizations need higher margins and smarter revenue strategies. According to Ganguly, AI can revolutionize how businesses model markets, understand buyer behavior, and communicate across channels—transforming traditional sales and marketing into precision-driven growth engines.
3. Legacy tech can’t support the future. As technologies like generative AI evolve rapidly, companies must continuously upgrade their platforms to stay relevant. Ganguly stresses that outdated infrastructures cannot support the scale or complexity of modern AI applications. Continuous modernization is key to integrating advanced technologies and unlocking new business opportunities.
"New challenges bring new opportunities", Ganguly concludes. "Organizations that embrace data and AI as core business capabilities—not just side projects—will be the ones that thrive in the next decade".